Future of AI in Dentistry: How SoftSmile Is Incorporating Advanced AI To Address The Needs of Patients
May 01, 2024
Future of AI in Dentistry: How SoftSmile Is Incorporating Advanced AI To Address The Needs of Patients
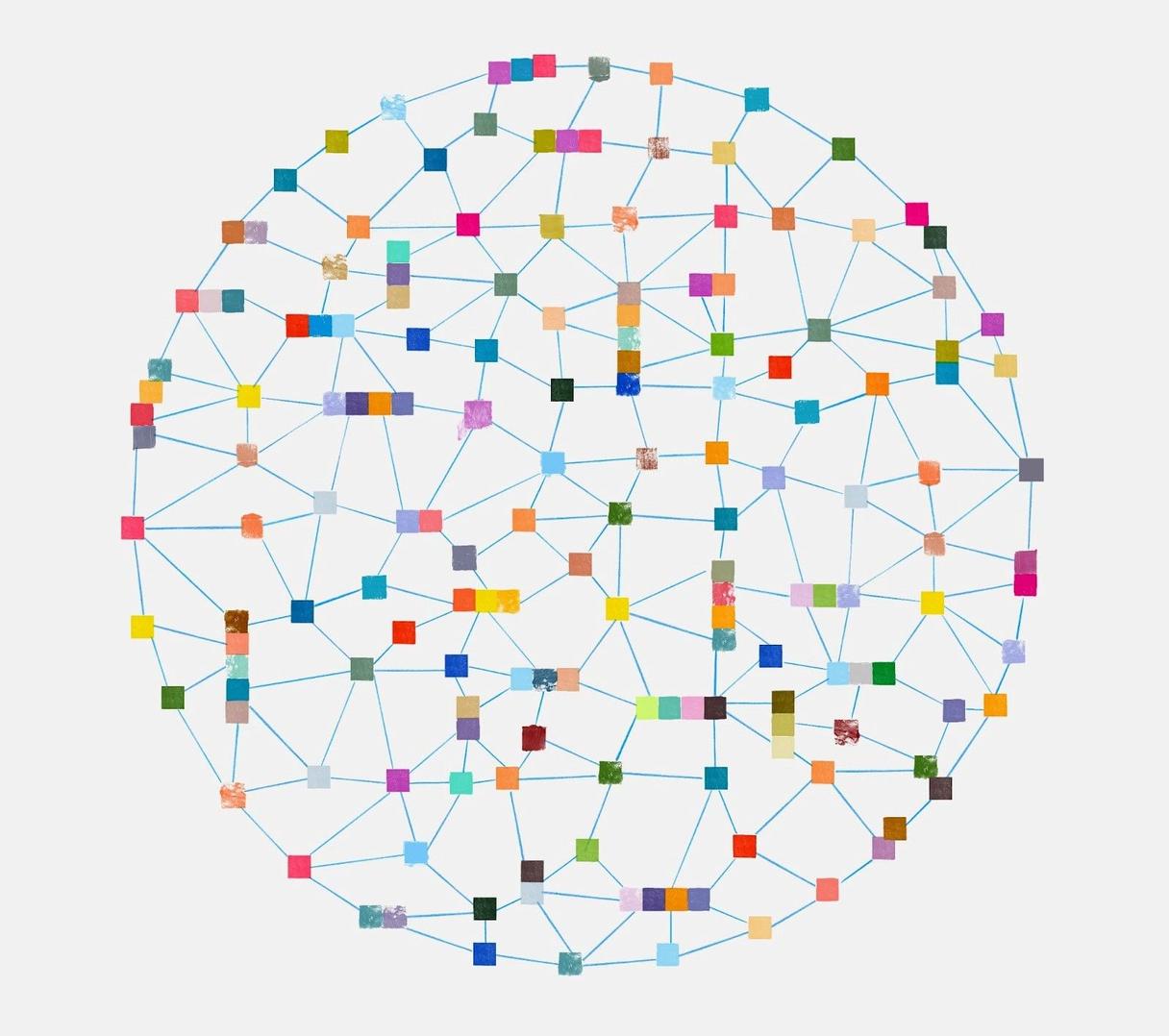
It is common for people to use the terms artificial intelligence (AI) and machine learning interchangeably. While SoftSmile’s digital treatment planning technology VISION incorporates both, it is important to understand how they are similar and how they are different.
We spoke with Damian Gerikhanov, SoftSmile’s Chief Engineer to better understand the concepts of AI and machine learning and how he and his team of engineers are using both AI and machine learning in VISION to improve how doctors can develop treatment plans faster, and more efficiently and improve patient care.
Can you explain the similarities and differences between AI and machine learning?
It’s important to note that not all AI involves machine learning. When people use the term 'machine learning' today, they are referring to something distinct from AI. The truth is that AI and machine learning offer different advantages, and it's essential not to dismiss the contributions of AI entirely because it provides substantial benefits in terms of usability, speed, and accuracy.
The truth is that machine learning is actually a part of AI. But they're not exactly the same. AI Is a more general term. When people say AI, what they really mean is machine learning which involves a lot of training data that the algorithm learns from.
Can you also explain how AI and machine learning are used in VISION?
Yes, of course! Let's start from the beginning. When raw scan data is uploaded to VISION, several processes occur that in the past would have required manual human intervention. One fundamental step is mesh cleaning, where the raw data undergoes cleaning using heuristics and artificial intelligence algorithms, which analyze geometries and remove unwanted elements.
Following that, the next critical step is segmentation, which determines which parts of the raw data represent different elements such as teeth, gingiva, or bone. Traditionally, older software required users to painstakingly draw lines to distinguish these tissues. Instead, modern solutions incorporate automated algorithms and AI to perform these tasks automatically.
The advanced AI responsible for segmentation typically employs heuristic algorithms, where human designers define the algorithm's purpose. In contrast, machine learning algorithms, as exemplified by Cone Beam Computed Tomography (CBCT) segmentation, undergo a training process.
During training, experts generate a dataset that captures their knowledge, which the algorithm learns from. This training takes weeks to months for dataset creation and days to weeks for the actual training. However, once trained, the algorithm can perform tasks in minutes that would take a human hours to complete.
Can you share a specific example of how advanced AI and machine learning have improved in VISION?
Let’s use CBCT as an example. CBCT segmentation, a highly intricate and time-consuming manual process, can now be fully automated, reducing the time required from hours to a mere two minutes.
Similarly, the task of Bolton analysis, marking teeth as present or absent and accounting for different tooth positions, used to involve extensive manual clicking. However, machine learning-based algorithms now automate this process, determining not only tooth absence but also identifying the specific teeth in place.
These machine-learning algorithms rely on data collected from thousands of anonymized cases where users manually perform these tasks. This data served as the training dataset for the algorithms.
Essentially, our approach involves initially allowing users to perform tasks manually and then leveraging the collected data to create algorithms that can partially or completely automate these tasks, thus reducing the burden on users.
How do neural networks factor into AI and machine learning?
You can build artificial intelligence using different types of things, right?
There are different algorithms and different methods of achieving that and machine learning is a family of algorithms that can give you artificial intelligence and the neural network is a subset of machine learning algorithms, which can give you a higher level of artificial intelligence.
So why do they call it a neural network? What we have in our brains are neurons that are connected. But when we talk about neural networks in the concept of AI, we're talking about artificial neural networks, which means we've built something in code that emulates real neurons in the biological sense.
For example, let’s look at a bird. We figure out how the wings work, and we build wings and then we automate the same way. We see how the bird’s brains work and then we build an analogy for the brain and it seems to work very well. Currently, the best AI is built with neural networks, because I mean, it just works.
How does SoftSmile’s use of AI and machine learning help to differentiate from competitors?
I think what really differentiates SoftSmile from other companies in this space is that we keep improving and updating VISION. I think one of those crucial recipes for success for SoftSmile is what I would describe as continuous learning. Also, having the algorithm self-learn is unique to SoftSmile. For instance, anytime the algorithm does something wrong, we collect that feedback from users and improve it over time.
Try out SoftSmile’s Digital Dental Treatment Planning Software: VISION.